|
Application of Dempster-Shafer Theory to Oil Monitoring
Authors :
X P Yan
Department of Marine Mech. Eng., Wuhan Transportation University, China
Y B Xie
Theory of Lubrication and Bearing Institute, Xi'an Jiaotong University, China
H L Xiao
Department of Marine Mech. Eng., Wuhan Transportation University, China
Abstract:
In order to solve the problem of diagnosing wear in tribosystem, evidence theory of Dampster-shafer is applied to realize the information fusion of multi-parameter in oil monitoring. Two diesel engines model 8NVD-48A were monitored under running condition by the oil monitoring methods such as spectrometric oil analysis, ferrographic monitoring, infrared spectrum analysis and oil quality testing. According to the results from the monitoring experiment, the types of worn parts and the relevant monitoring characteristic are summarized. The worn parts are mainly pointed to scoring, seizure and corrosion between piston (or piston ring) and cylinder liner; scratching, seizure, spalling and corrosion in gear; pitting, seizure and fatigue in gear. Based on the experience and rules of some experts, basic probability assignment for Dampster-shafer is given out, and the weights of different oil monitoring for different wear failures are also determined. The calculation of combination rule of seizure between piston and cylinder liner is also advanced by using D-S method. The analysis and calculation show that principle of data fusion is useful for information process in oil monitoring.
1. Introduction
Tribological failures result from tribological behaviour of rubbing pairs in a machine, and the failure is different with different machines because the applied load, operation environment, working temperature, lubricating condition and operation period are changeable. Oil monitoring can be used to diagnose the tribological failures. Generally, through a sample from lubricating system in a monitored machine, the tribological failures are identified due to quality changes of the lubricants and wear particles analysis. But it is not easy to reach this goal, for the reason of the features of tribological failure being changed with the changes of the operation hours and system's properties. The monitoring experiment showed that the feature from a single monitor is limited, which is influenced not only by the principle of some oil monitors, but also by the complexity of tribological failure. Recently, more and more engineers know that it is useful to add the oil monitors in order to obtain much original information of tribological failure and it is most important to develop the data processing method. It followed that the tribological failure types can be effectively diagnosed due to the systematic use of oil monitors. All of the first, it is the key step how to fusion the data from the different oil monitoring techniques. When the analyst writes the diagnostic report, he should deal with the monitoring data according to some mathematics pattern, and he will effectively use the data in this way. This is why some researchers and engineers applied the information fusion into oil monitoring. Information fusion (or data fusion) deals with the synergistic combination of information made available by various knowledge sources such as sensors, in order to provide a better understanding of a given scene (Waltz and Linas, 1990; Abidi and Gonzalez, 1992; Loera, Thompson and Akbar, 1992). Oil monitoring require a way which matches information gathered by various knowledge sources and generate the useful diagnostic rules through the information features. For the tribological failure, we often refer two types of faults, one is wear of parts and another is lubricant deterioration. We should establish some mathematics model that can be used to classify this two aspects of the tribological failures. For this goal, we chose the data fusion to process the data from different oil monitoring techniques such as SOA, ferrograghic monitoring, infra-red spectrum analysis and oil quality testing. For the oil monitoring experience, it is more different to determine the types of tribological failures. So we use the evidence theory to process the data from different monitors. After summarizing the information features and calculating the basic probability assignment, the combination evidence is given out. In this way, the accuracy of failure diagnosis is increased (Yan, 1997).
2. Tribological Failure Types and Their Features
The monitoring experiment is conducted on two 8NVD48A-2u marine diesel engines that were mounted in a passenger ship voyaging in Yanzi River. According to the monitoring results and some other monitoring examples of the same type engine, the wear types of parts and their features from oil monitoring are summarized and listed in Table 1. For the tribosystem in diesel engine, wears of parts are the main tribological failure which include scoring, seizure and corrosion between piston and cylinder liner; scratching, seizure, spalling and corrosion in bearings; and pitting, scuffing and spalling in gear. From Table 1, we found that most kinds of wear are revealed from the various oil monitoring methods, but some kinds of wear are given out the same signals from one special monitoring method. In the other words, some oil monitoring methods are given no sense for some failures. For example, both infra-red spectrum analysis and oil quality testing are no remark about scoring between piston and cylinder liner or pitting in gear. It has therefore displayed that it is necessary to apply data fusion technique to information processing in oil monitoring so that the diagnostic results are successfully obtained by the analyst.
3. Dempster-shafer Theory
3.1 Basic Probability Assignment
In the D-S theory, the set of all possible outcomes in a random experiments is called the frame of discernment (FOD), usually denoted by q.
The ( ) subsets of q are called propositions, and probability masses are assigned to propositions, i.e., to subsets of q.
The interpretation to be given to the probability mass assigned to a subset of q is that the mass is free to move to any element of the subset. Under this interpretation, the probability mass assigned to q represents ignorance, since this mass may move to any element of the entire FOD. When a source of evidence assigns probability masses to the propositions represented by subsets of q, the resulting function is called a basic probability assignment (bpa).
Formally, a bpa is function: where
Subsets of q
that are assigned non zero probability mass are said to be focal elements of m. The core of m is the union of its focal elements. A belief function, Bel(A),over q
is defined by
(1)
Table 1 Wear types of main parts and information characteristics in oil monitoring
Number |
Parts Name |
Failures Type |
Information Characteristic from Different Oil Monitor |
SOA |
Ferrographic monitoring |
Infrared* |
Quality** |
1 |
Piston & Cylinder Liner |
Scoring |
Abnormal in concentration of ferrous-element |
Small cast iron cutting wear particle |
Soot Increases |
Benzene insoluble increases |
Seizure |
Abnormal in concentration of both ferrous and nonferrous element |
Cast iron and aluminum severe sliding wear particle with a rough surface |
|
TAN increases |
Corrosion |
Abnormal in concentration of ferrous-element |
Corrosive wear debris |
Sulfation increases |
|
2 |
Bearing |
Scratching |
Abnormal in concentration of nonferrous element;Silicon increase |
Nonferrous cutting wear particles |
|
Benzene insoluble increases |
Seizure |
Abnormal in concentration of nonferrous element |
Nonferrous metal wear particles with oxidation; Black oxides of iron |
Oxidation increases |
Viscosity increases |
Spalling |
Abnormal in concentration of nonferrous element |
Nonferrous metal fatigue particle |
|
|
Corrosion |
Abnormal in concentration of nonferrous element |
Corrosive wear debris |
Sulfation increases |
TAN increases |
3 |
Gear |
Pitting |
Abnormal in concentration of ferrous-element |
Steel rubbing and fatigue wear particle |
|
|
Scuffing |
Abnormal in concentration of ferrous-element |
Steel severe sliding wear particles with striations |
Oxidation increases |
Benzene insoluble increases |
Spalling |
Abnormal in concentration of nonferrous element |
Steel severe sliding wear particles with a rough surface |
Antiwear additive reduces |
|
*Infrared spectrum analysis; **Oil quality testing
3.2 D-S Combination Rule
Assume that belief function are assigned to independent sources of evidence in same frame of discernment (i.e., ), and the relevant basic probability assignment is m1, m2, ., mn, then according to Dempster's rule of combination, the new belief function Bel(A) and basic probability assignment m(A) may be yielded via
(2)
(3)
The formula is commonly called Dempster's orthogonal sum. Here, we use the notation Å
to represent the combination.
If there is a pose/identity hypotheses, assume that the hypotheses A supporting by each source is respectively m1(A), m2(A), ., mn(A), and the hypotheses A refusing by the each source is respectively m1(q), m2(q),.,mn(q), where:
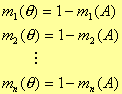
We have combination rule:
(4)
D-S Combination Rule for Diagnosing Tribological Failures
The oil monitoring experiment showed that we can get some features from the various monitors, then the features are used to identify the failure in monitored machine, so it is very important to integrate and match those features. So the goal of applying Dempster-shafer into tribological failure diagnosis is to synthesis the features from different oil monitors, and to get a combination rule. After the combination rule is reached, we will get the more accurate analysis results.
As mentioned above, it is critical to determine a basic probability assignment when we use the D-S model to match the information. Generally, the basic probability assignment is closely relative to the data type and special objective. When we established the basic probability assignment, we acquired knowledge from some experts who got more useful information on oil monitoring. We designed some knowledge definition and collecting tables and distributed those tables to the experts in Universities, factories and research organizations. From the filled tables, the basic probability assignment for worn parts is summarized and listed in Table 2.
Table 2 Basic probability assignment of tribological failure in oil monitoring
Number | Features in oil monitoring* | Piston & cylinder liner | Bearing | Gear |
Scoring | Seizure | Corrosion | Scratching | Seizure | Spalling | Corrosion | Pitting | Scuffing | Spalling |
1 | A | 0.3 | | 0.5 | | | | | 0.3 | 0.3 | 0.3 |
2 | B | | 0.4 | | | | | | | | |
3 | C | | | | | 0.3 | 0.2 | 0.5 | | | |
4 | D | | | | 0.5 | | | | | | |
5 | E | 0.5 | | | | | | | | | |
6 | F | | 0.7 | | | | | | | | |
7 | G | | | 0.3 | | | | 0.3 | | | |
8 | H | | | | 0.4 | | | | | | |
9 | I | | | | | 0.5 | | | | | |
10 | J | | | | | | 0.5 | | | | |
11 | K | | | | | | | | 0.4 | | |
12 | L | | | | | | | | | 0.5 | |
13 | M | | | | | | | | | | 0.3 |
14 | N | | 0.3 | | | | | | | | |
15 | O | | | 0.3 | | | | 0.3 | | | |
16 | P | | | | | 0.3 | | | | | |
17 | R | | | | | | | | | | 0.3 |
18 | S | | 0.3 | | 0.3 | | | | | 0.3 | |
19 | T | | | 0.3 | | | | 0.3 | | | |
20 | U | | | | | 0.3 | | | | | |
The meaning of letter in Table 2 is listed as following:
- Abnormal in concentration of ferrous element
- Abnormal in concentration of both ferrous and nonferrous element
- Abnormal in concentration of nonferrous element;
- Abnormal in concentration of nonferrous element; Silicon increases
- Small cast iron cutting wear particle
- Cast iron and aluminum severe sliding wear particle with a rough surface; Black oxides of iron
- Corrosive wear debris
- Nonferrous cutting wear particles
- Nonferrous metal wear particles with oxidation; Black oxides of iron
- Nonferrous metal fatigue particle
- Steel rubbing wear particle and fatigue particle
- Steel severe sliding wear particles with striations; Black oxides of iron
- Steel severe sliding wear particles with a rough surface
- Soot increase
- Sulfation increase
- Oxidation increases
- Antiwear additive reduces
- Benzene insoluble increases
- TAN increases
- Viscosity increases
It is possible to calculate the D-S combination rule according to the value in Table 2. For example, the failure of seizure generated between piston and cylinder liner in a diesel engine was selected to show the calculation of D-S combination rule. From Table 2, we see that the different supporting values are generated from the various oil monitors. The combination rule is calculated as followed. According to Table 2, the basic probability assignment for SOA, ferrographic monitoring, infra-red spectrum analysis and oil quality testing are respectively listed here,
On the base of Formula (4), obtained:
If one oil monitor is no signal on the feature listed in Table 2, the combination rule will be decreased. For instance, for the seizure in diesel engine, maybe the infra-red spectrum analysis doesn't displayed the increase of soot, then the combination rule will be:
To compare the calculation above, it is clearly showed that the more information is collected, the high reliability of diagnosis result will be. The combination rules of tribological failures for 8NVD48A-2u marine diesel engine are shown in Table 3. There are 11 possibilities and the accuracy of diagnosis is increased as the oil monitors added.
Table 3 Dempster-shafer combination rule of tribological failure
Number | Oil monitoring methods | Piston & cylinder liner | Bearing | Gear |
Scoring | Seizure | Corrosion | Scratching | Seizure | Spalling | Corrosion | Pitting | Scuffing | Spalling |
1 | SOA; Ferrography | 0.65 | 0.82 | 0.65 | 0.70 | 0.58 | 0.60 | 0.65 | 0.65 | 0.65 | 0.65 |
2 | SOA; Infrared spectrum analysis | | 0.58 | 0.65 | | 0.51 | | 0.65 | | 0.51 | |
3 | SOA; Oil quality testing | | 0.58 | 0.65 | 0.65 | 0.51 | | 0.65 | | 0.51 | |
4 | Ferrography; Infra-red spectrum analysis | | 0.79 | 0.51 | | 0.65 | | 0.51 | | | 0.65 |
5 | Ferrography; Oil quality testing | | 0.79 | 0.51 | 0.58 | 0.65 | | 0.51 | | 0.65 | |
6 | Infrared spectrum analysis; Oil quality testing | | 0.51 | 0.51 | | 0.51 | | 0.51 | | | |
7 | SOA; Ferrography; Infrared spectrum analysis | | 0.874 | 0.755 | | 0.755 | | 0.755 | | | 0.755 |
8 | SOA; Ferrography; Oil quality testing | | 0.874 | 0.755 | | 0.790 | | 0.755 | | | 0.755 |
9 | SOA; Infrared spectrum; Oil quality testing | | 0.706 | 0.755 | | 0.657 | | 0.755 | | | |
10 | Ferrography; Infrared spectrum analysis; Oil quality testing | | 0.853 | 0.657 | | 0.755 | | 0.657 | | | |
11 | SOA; Ferrography; Infrared spectrum analysis; Oil quality testing | | 0.9118 | 0.8285 | | 0.8285 | | 0.8285 | | | |
5. Conclusions
- Based on oil monitoring results of 8NVD48A-2u marine diesel engine under running condition and combined with some diagnosis cases collected from different applications, the information features of worn parts from oil monitoring are summarized.
- In discussion of Dempster-shafer evidence theory, the use and meaning of basic probability assignment and belief function are introduced, then the calculating equation of combination rule on diagnosing tribological failure is developed.
- The combination rule from different oil monitoring methods is obtained by the calculating example. The research states that the more information can be gained from the used oil sample, the high accuracy of diagnosing failure will be.
References
Waltz, E and Linas, J (1990), Multisensor data fusion. Artech, Norwood, Massachusetts
Abidi, M A and Gonzalez, R C (1992), Data fusion in robotics and machine intelligence. Academic Press, Inc. USA
Loera R P, Thompson W E and Akbar S A (1992), Multilevel distributed fusion of multisensors data. SPIE ,1699:127
Yan, X P (1997), Research on oil monitoring and information fusion for tribosystem. Doctor thesis, Xi'an Jiaotong University, China
Copyright 1996-2009, The Plant Maintenance Resource Center . All Rights Reserved.
Revised: Thursday, 08-Oct-2015 11:52:03 AEDT
Privacy Policy
|
|